
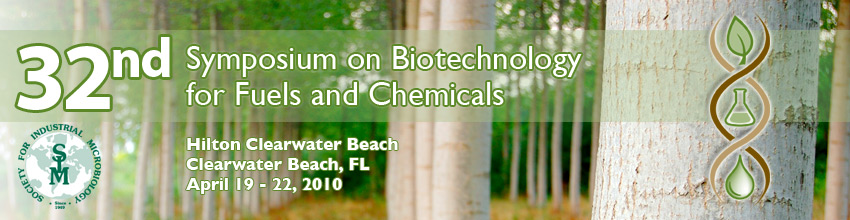
Monday, April 19, 2010
2-20
Comparison of methods for integrating poplar metabolite and transcriptomic data
David Astling1, Peter Graf2, Kofi Adragni2, Jinsuk Lee2, and Mark Davis1. (1) National Bioenergy Center and BioEnergy Science Center, National Renewable Energy Laboratory, 1617 Cole Boulevard, Golden, CO 80401, (2) Scientific Computing Center, National Renewable Energy Laboratory, 1617 Cole Boulevard, Golden, CO 80401
Biomass derived from plants such as poplar has been identified as one of the key feedstocks for the development of next generation biofuels. In order to convert woody biomass to fermentable sugars we must have a good understanding of the genetic and metabolic processes involved in cell wall biosynthesis. We have used high-throughput techniques such as microarrays and molecular beam mass spectrometry (MBMS) to elucidate metabolic pathways and signal transduction cascades involved in altering cell wall chemistry. To integrate and draw correlations amongst these large py-MBMS and microarray data sets, we are exploring three published multivariate statistical methods; O2PLS, Principal Fitted Components and Elastic Net. i) The O2PLS method, a 2-way Orthogonal correction to Partial Least Squares (PLS) regression, is ideally suited for analyzing information across multiple platforms because it separates the systematic orthogonal variation from the joint covariation for both datasets. ii) The Principal Fitted Components (PFC) method shares some similarity to the O2PLS method by estimating the joint variation in both sets of data. In addition the PFC method can be used to obtain a sufficient reduction of the data, i.e. fewer number of variables that can be used to simplify further analysis. iii) And finally the Elastic Net is an alternative method for obtaining correlations between each data set. We are currently comparing and evaluating the results of each method with data from several projects.
See more of Poster Session One Sponsored by Genencor
See more of General Submissions
See more of The 32nd Symposium on Biotechnology for Fuels and Chemicals (April 19-22, 2010)
See more of General Submissions
See more of The 32nd Symposium on Biotechnology for Fuels and Chemicals (April 19-22, 2010)