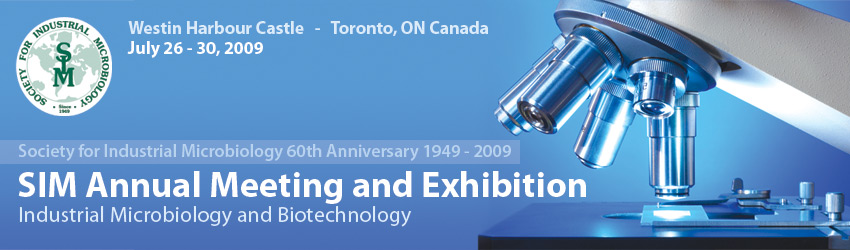
Tuesday, July 28, 2009 - 10:30 AM
S75
Interfacing mathematical models of cells with metabolic data
In the another example, fitness profiling data from growth competition experiments between strains of different genetic backgrounds were used to refine the metabolic model of Escherichia coli. Constraint-based models of metabolism seldom incorporate capacity constraints on intracellular fluxes due to lack of kinetic data. Here, the optimal capacity constraint identification (OCCI) algorithm was developed to identify capacity constraints necessary for accurately predicting experimental fitness profiles. In a case study, fitness profiles from 14 different genetic backgrounds in the same growth environment were used to identify capacity constraints in central metabolism of E. coli. This algorithm can be readily extended to handle Phenotype Micro-array data from multiple growth environments.