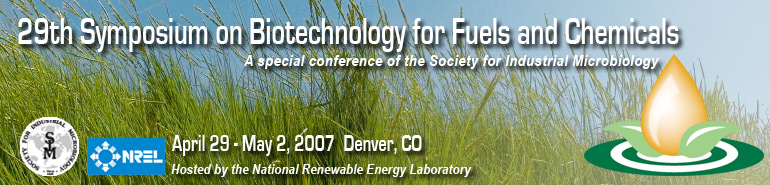
Monday, April 30, 2007
6-16
Kinetic modeling and parameter estimation in a pseudo-expanded bed tower bioreactor for bioethanol production
Elmer Ccopa Rivera1, Aline C. Costa1, Silvio R. Andrietta2, Betânia H. Lunelli1, and Rubens Maciel Filho3. (1) School of Chemical Engineering, State University of Campinas, Laboratory of Optimization, Design and Advanced Control, Cidade Universitária Zeferino Vaz, CEP: 13081-970, Campinas - SP, Brazil, (2) Multidisciplinary Center for Chemical, Biological and Agricultural Research, State University of Campinas, P.O. Box 6171, CEP: 13081-970, Campinas, Brazil, (3) Department of Chemical Process – School of Chemical Engineering, State University of Campinas, Cidade Universitária, P.O. Box 6066, Campinas, Brazil
Estimation of kinetic parameters of deterministic models is usually complex, mainly due to non-linearities, great number of parameters and interactions among them. In biochemical engineering, the most classical method involves the mathematical estimation of model parameters based on the minimization of some cost function built-up with the parameters to be estimated. Several kinetic models have been proposed for the alcoholic fermentation process. Many techniques are available for minimizing the error of estimation, generally methods based on local gradient search.
In this work, a systematic method to support the building of bioprocess models through the used of different optimization techniques, is presented. The method was applied to a pseudo-expanded bed tower bioreactor for bioethanol production with self-immobilized cell of Saccharomyces Cerevisiae. Specifically, the step-by-step procedure used to the estimation problem is detailed as follows. The first step is to estimate initial values for all parameters in the model. Subsequently, the potential of global searching of Real-Coded Genetic Algorithm (RGA) was applied for simultaneous estimation of the parameters. In the third step the most significant parameters were identified using Placket–Burman (PB) design. Finally, the Quasi-Newton algorithm (QN) was used for optimization of the most significant parameters, near the global optimum region, as the initial values were already determined by the RGA global searching algorithm. The results have shown that the performance of the model to describe the experimental data is improved using the proposed method (RGA-PB-QN) in comparison with a model whose parameters were only optimized by RGA.
In this work, a systematic method to support the building of bioprocess models through the used of different optimization techniques, is presented. The method was applied to a pseudo-expanded bed tower bioreactor for bioethanol production with self-immobilized cell of Saccharomyces Cerevisiae. Specifically, the step-by-step procedure used to the estimation problem is detailed as follows. The first step is to estimate initial values for all parameters in the model. Subsequently, the potential of global searching of Real-Coded Genetic Algorithm (RGA) was applied for simultaneous estimation of the parameters. In the third step the most significant parameters were identified using Placket–Burman (PB) design. Finally, the Quasi-Newton algorithm (QN) was used for optimization of the most significant parameters, near the global optimum region, as the initial values were already determined by the RGA global searching algorithm. The results have shown that the performance of the model to describe the experimental data is improved using the proposed method (RGA-PB-QN) in comparison with a model whose parameters were only optimized by RGA.
See more of Poster Session 2
See more of General Submissions
See more of The 29th Symposium on Biotechnology for Fuels and Chemicals (April 29 - May 2, 2007)
See more of General Submissions
See more of The 29th Symposium on Biotechnology for Fuels and Chemicals (April 29 - May 2, 2007)